Enhancing Sleep Quality: The Role of AI in Sleep Monitoring and Analysis
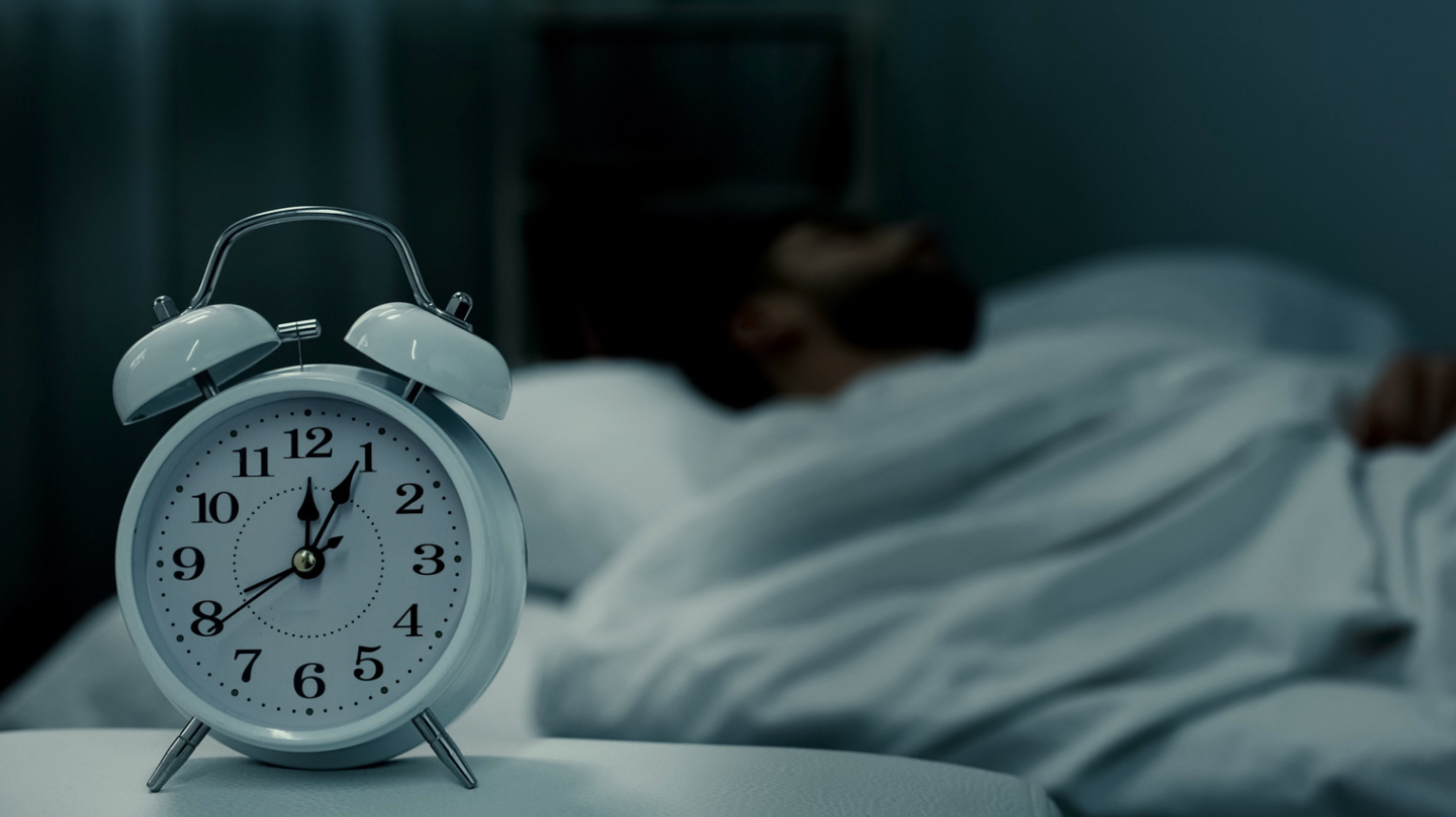
Sleep Monitoring
Tallywell monitors your sleeping patterns through the HealthKit application of the Apple watch. It advises on how much sleep you need to get, on average, to achieve a better quality of life.
In general, AI can be used to monitor sleep through a combination of hardware sensors, data collection, and advanced algorithms for data analysis.
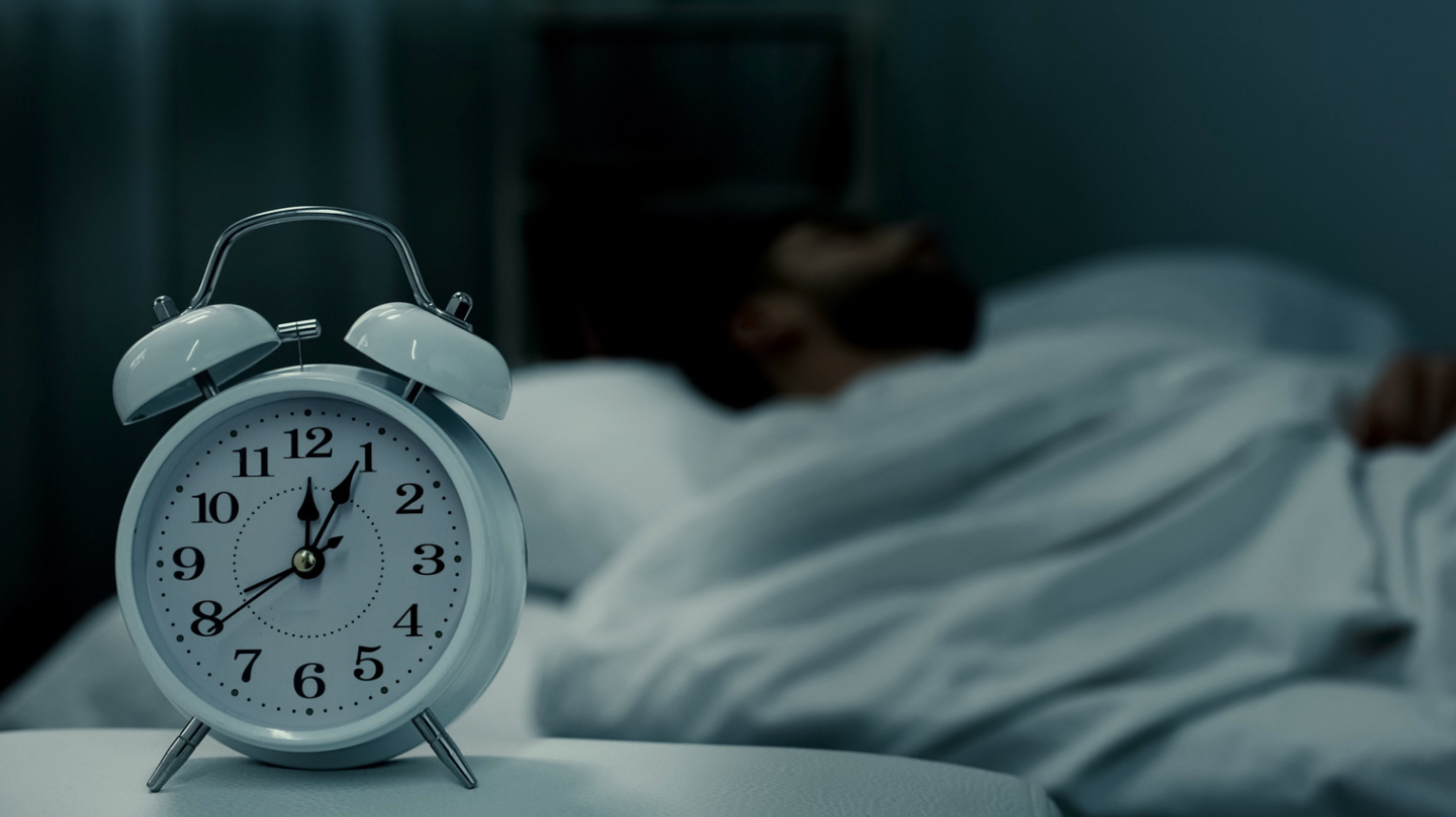
This is how it could work in practice:
First and foremost, the hardware collects a person's sleep.
This data is usually gathered in wearable dev
ices, smartphones and environmental sensors in the bedroom attached to mattresses and pillows.
Typical sensors are heart rate monitors, temperature sensors, microphones, and ambient light sensors.
The sensors gather all data all night, that's important for consistency purposes.
Data acquisition may include monitoring movement, heart rate, body temperature, noise levels, and other relevant parameters.
Data collected from sensors may be subject to smoothing, gap filling, filtering, noise reduction, and data normalization.
It is important to extract features from the preprocessed data that have predictive relevance such as heart rate variability, sleep posture changes, snoring sounds, or body movements can be extracted.
In general, predictive analytics such machine learning, deep learning, or even simple statistical methods can be used to extract and analyze the features.
These algorithms are trained on large datasets to learn patterns associated with different sleep patterns and disorders and, more specifically, sleep quality.
As it is well known, there are different sleep stages such as deep sleep, REM (rapid eye movement) sleep, and light sleep.
One of the primary goals of sleep monitoring is to classify sleep stages during the night using AI models and the extracted set of features
Sleep quality depends on a series of factors such as sleep duration, the number of awakenings, sleep efficiency (the ratio of time asleep to time in bed), and the presence of sleep disturbances.

In addition, the sensors couple with the machine learning models can detect sleep disorders like sleep apnea, insomnia, or restless leg syndrome by looking at specific patterns and anomalies in the collected data.
In the case of sleep apnea, it can look at the breathing patterns.
AI applications such as tallywell often provide personalized insights and recommendations based on the sleep data analysis.
These recommendations may include adjustments to sleep schedules and exercise.
As in tallywell, the results of the sleep monitoring are presented to users in the form of data visualizations, reports, or notifications through a smartphone app , through the Apple watch itself. or even a web site.
On the basis of these visual reports, customers can track their sleep habits over time and make informed decisions to improve sleep quality.
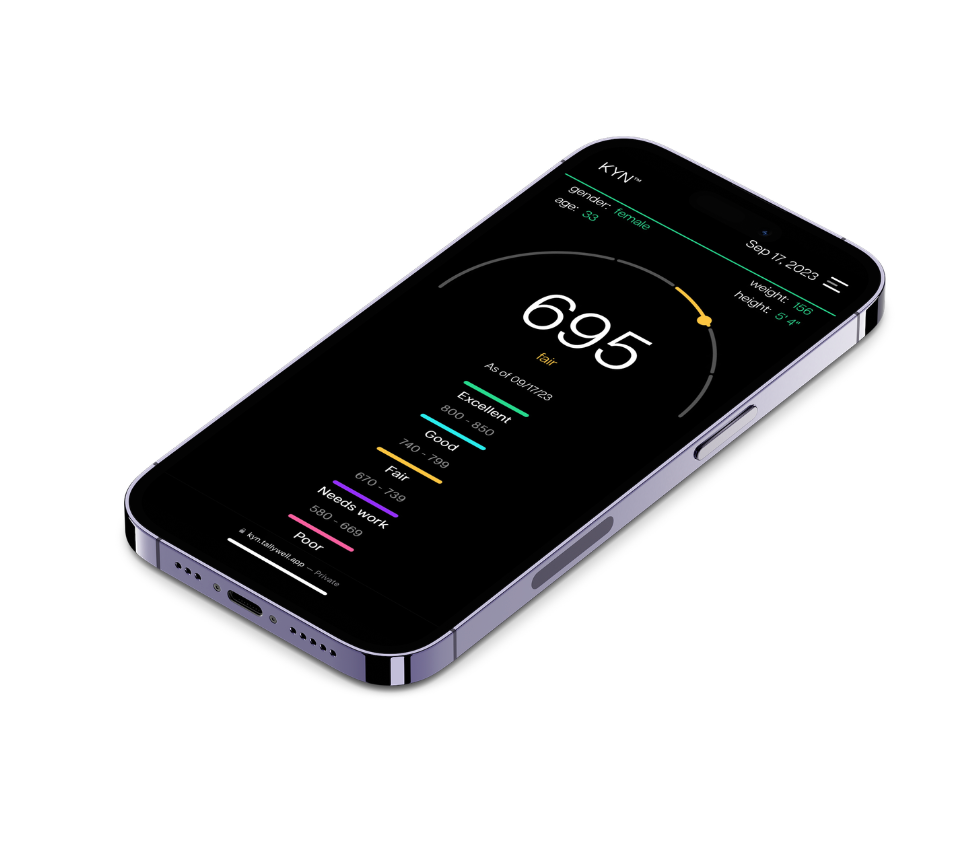
Tallywell and many other sleep apps offer continuous sleep monitoring, allowing users to track changes in their sleep patterns and adjust their habits accordingly.
In addition, tallywell’s sleep monitoring system integrate sleep data with other health-related data, such as number of daily steps, BMI, and heart rates at rest, to provide a more holistic view of overall well-being.
To summarize, AI applications for sleep monitoring employ sensor data, machine learning algorithms, and other data analysis techniques to generate insights into sleep patterns, quality, and potential sleep disorders.
These applications allow individuals to make better and informed decisions about their sleep habits and improve their overall sleep health.